How to code and tag qualitative data to unlock research insights
#Tagging tips from #PeopleNerds.
While quantitative data is simple and straightforward, researchers using qualitative methods enjoy a richer data set—but one that is not always rigidly categorized or easy to parse. If researchers are more used to structured data like survey results or usability tests, qualitative data can seem overwhelming. How do you translate open-ended responses, photos, video clips or even whole interview transcripts into themes, insights and even charts?
Enter tags. In research, tagging, or coding, can be a bit like a decryption key—find the right tags, and the larger themes in your data will start to rise to the surface. Coding also facilitates collaboration and teamwork, flags problem areas where researchers may be making assumptions, and helps clients understand the volume and depth of your research and analysis.
As Sara McGuyer, Principal at Yes and Yonder, told us: “I like to use a mix of methods to see a design challenge from multiple angles and typically collect quite a bit of data. Without some sort of coding system, that much qualitative data can get overwhelming, messy, and really hard to process. The visual cues of using color and symbols make it easier to see the big picture at a glance, which makes it easier to introduce the data to people who haven't been part of the research efforts.”
So what makes for a good tag? Think short, descriptive labels that can be used to describe different sets of qualitative data. They can be used to describe the content of an interview, or the context. In a digital context (with remote research platforms like dscout), or an analog one (hello, wall of sticky notes). And if your project is complex, tagging can be an essential tool for categorizing, quantifying and sorting the data you’ve collected—and a secret weapon in getting to insights you might otherwise miss.
Read on for more insights from Sara and other People Nerds to learn how you can make tagging go to work for you.
1. Decide on an approach: deductive or inductive.
Just like your approach to research overall, your coding can be deductive or inductive. If you’re using a deductive coding method, you’ll develop your codes before parsing your data. For an inductive method, you’ll refrain from coming up with codes until after you’ve looked through your data, letting the codes reveal themselves. Both methods have advantages and drawbacks, and often, projects will use a combination of both.
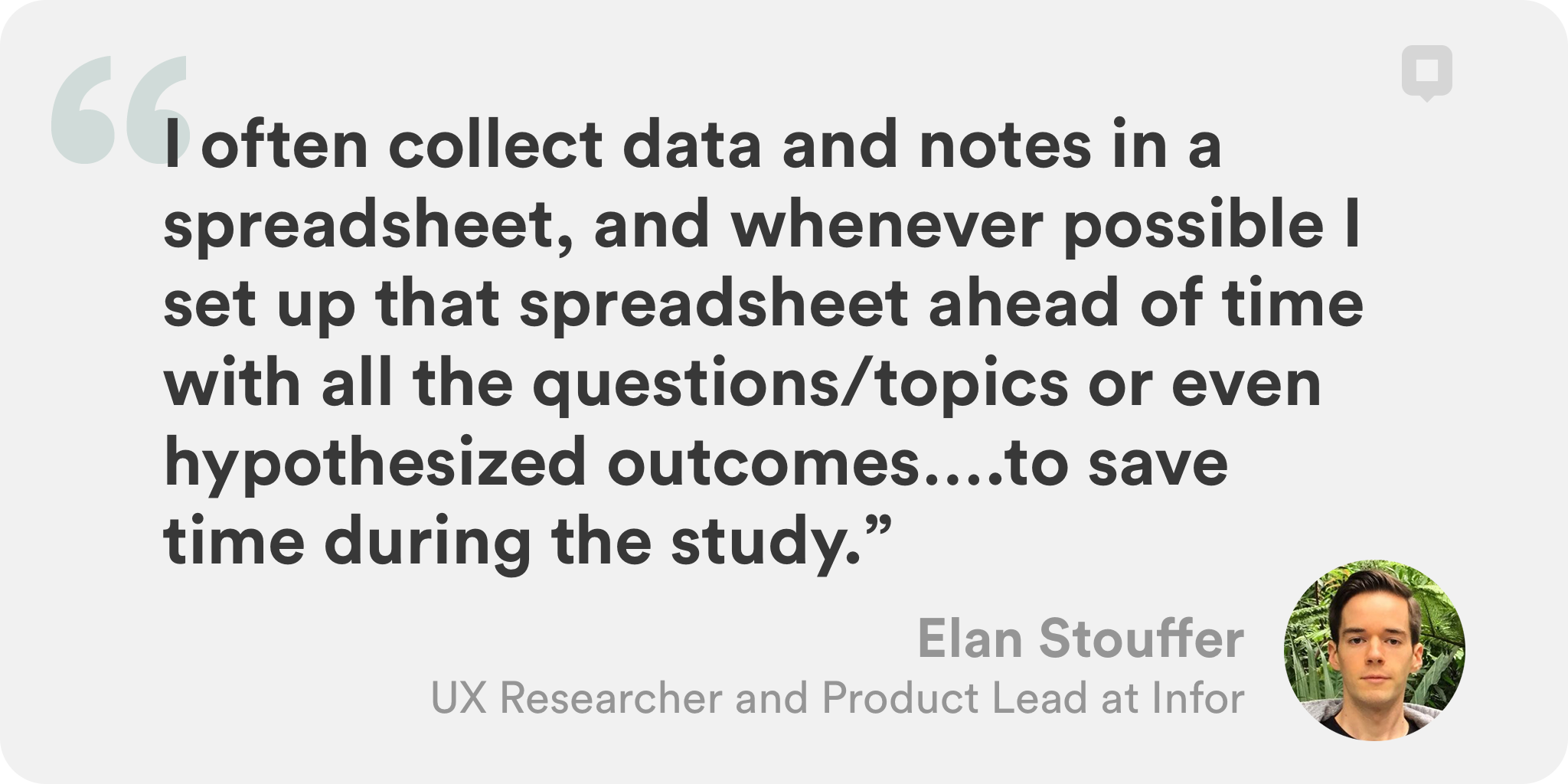
Elan Stouffer, a UX Researcher and Product Lead at Infor, likes the speed and efficiency of a deductive method: “I often collect data and notes in a spreadsheet, and whenever possible I set up that spreadsheet ahead of time with all the questions/topics or even hypothesized outcomes. Whatever we can do ahead of time will save time during the study.”
Erika Spear, Ph.D., a Cyber-Social and UX Researcher at Project UX, says coming up with an idea of your tags beforehand can also help steer your interviews toward the data that clients want to see—a method that can also expedite things for researchers on a deadline. “If I already talked to stakeholders and read about other's research beforehand, I'll have a rough idea of what the codes will look like. I can come up with a rough list of codes even before the interviews and try to prompt my participants to talk more about the key things the stakeholders want to learn.”
Jess Sand, UX Content Strategy Consultant, often utilizes an inductive method in the early stages of research—especially when trying to understand an audience’s mental models. “I’ll often jot statements and thoughts/ideas down on sticky notes, and slap them up on the wall as I transcribe,” Sand says. “By the end of the interview, it’s normal to have some very rough groupings on the wall.”
She adds that while you can go into a project with an idea of what codes will be, you can’t really know until you’ve got the data in front of you—following grounded theory. “I’m about to do some contextual inquiry with a group of “citizen scientists” who are collecting air quality data in their neighborhood. My guess is that the code I use is likely to include themes around communities (e.g. people, places, experiences), as well as behaviors (maybe things like communicating, traveling/commuting, shopping, studying, etc), and emotions (e.g. fear, pride, anger, distrust, love, etc). This is just a guess—the interviews themselves will give me the appropriate code.”
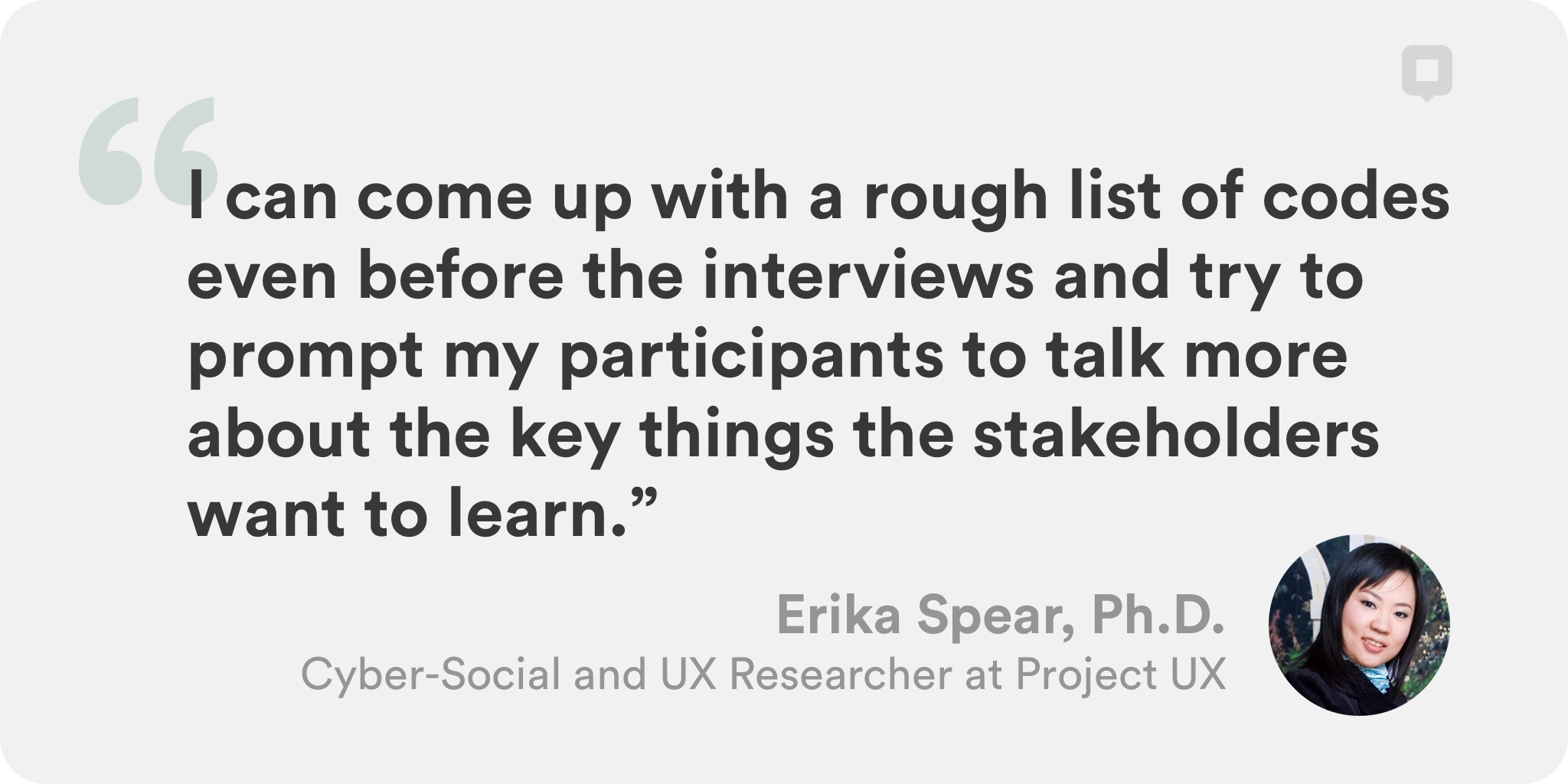
2. Start with basic, descriptive themes that appear regularly.
At the outset, you’ll want to stick to the basics: words or phrases that participants use repeatedly, or anything objective that you can pull out from your research. These are “Descriptive Tags” and are often situational, such as locations or actions.
If you want to get an overall sense of the direction your data might go in, one method is to pick out a few “Power respondents”—people who gave robust answers to your questions or thoughtfully answered a specific question that’s central to your inquiry. If you take a closer look at a handful of power respondents first, it can give you a good sense of what your overall data may trend toward.
That said, Hareth Al-Janabi from the Institute of Applied Health Research emphasizes that it is important to keep an open mind at the initial coding stage. “It can be easy to get stuck into a mindset of picking a single 'type' of code. Keep the research question in mind, but be creative about what is coded and what codes you assign. Early on in the coding process, it's better to stick closely to the words and phrases used by the participants—you don't want to be too deductive at the beginning and risk misunderstanding what the participant is getting at. However, as your coding and analysis progresses, it's good to identify more analytical codes that capture the essence of concepts that have been verbalized perhaps in different ways by different participants.”
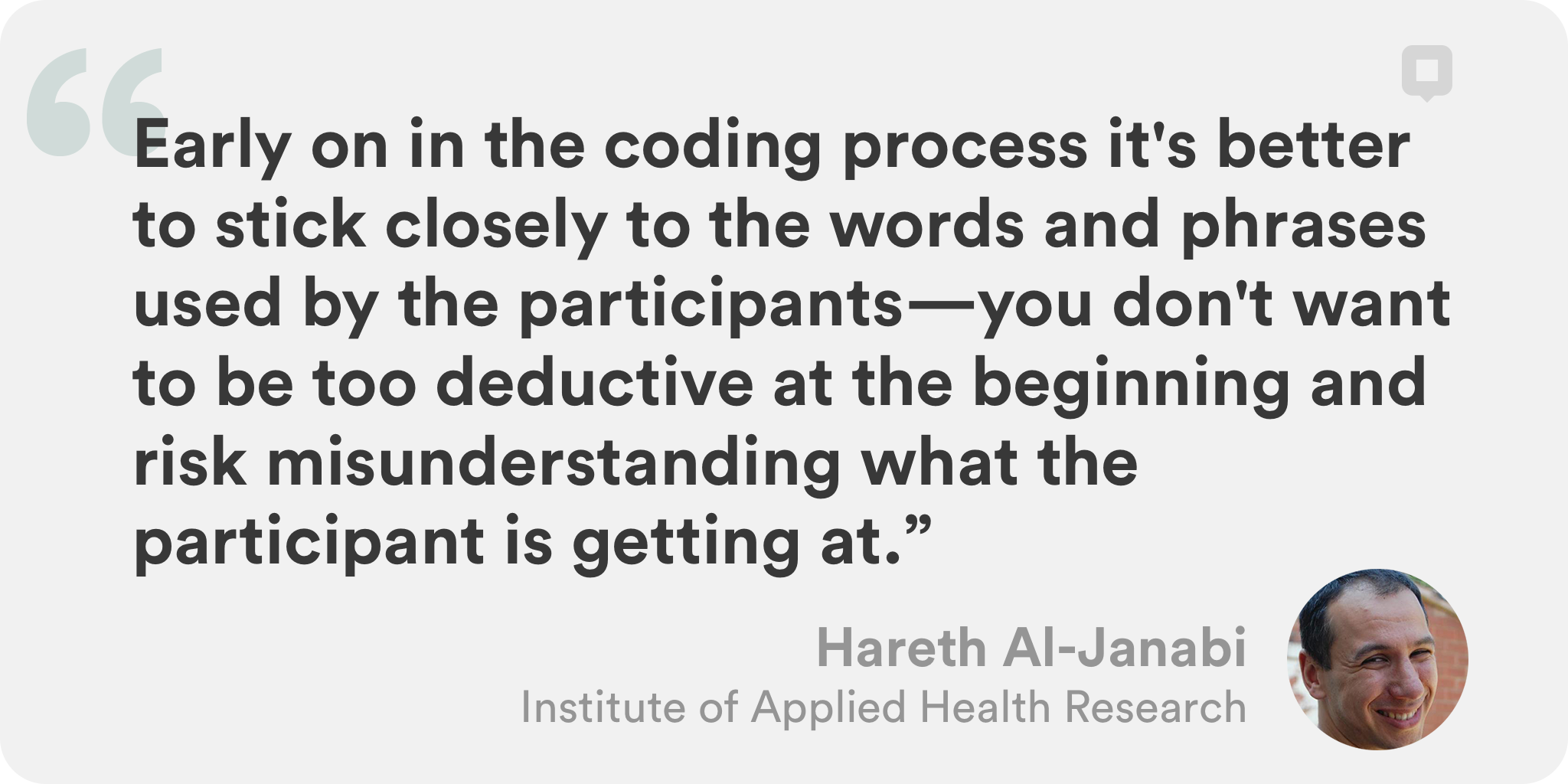
Erika Spear cites W.F. Owen’s criteria for thematic analysis—repetition, recurrence, and forcefulness—as her go-to for deciphering data. The first two criteria of this method (stay tuned for more on the third) include tags in the “descriptive” category.
“Repetition means if a participant repeatedly mentions something, then it must be important for him/her,” says Spear. “Recurrence is how many times the similar codes appear in all of your data.”
It’s a good method to follow as you’re taking the first or second pass through your data.
There will be tons of things you can tag descriptively—digital or online research tools often can auto-tag submissions once you’ve decided on your keyword(s). The rich data can feel overwhelming when getting started, though, so be sure to focus on areas that are likely to be relevant to your research question.
3. Then add the intangibles: emotion, emphasis, and other contextual clues.
As Hareth Al-Janabi points out, a tag “can be anything, as long as it relates to the research question.”
For many researchers, that means noting the subtext and emotion captured in a subject’s response, or finding the thematic tags that help quantify some of the deeper themes. Elan Stouffer looks for signs of surprise, confusion, or frustration. He’ll also make a note if someone liked or disliked something, or if they had a strong emotional reaction.
Erika Spear says this is where the third element of Owen’s method comes in: forcefulness. “Forcefulness requires you to evaluate emotional engagement during the interview process,” she says. “Some powerful stories could be short, or only appear once in your data, but the forcefulness gives it weight.”
But reading the subtext doesn’t just mean logging emotion. Sara McGuyer has developed an intricate system for flagging non-verbal elements: “I add notations for things like the source or quality of the data point. If a quote came from my fifth interview, I'll tag that. If an item is repeated frequently, I'll make a note of the strength of repetition. Or an exclamation point to note I am making an inference about something. Some research may require a different kind of coding, and I think it's ok to devise your own system.”
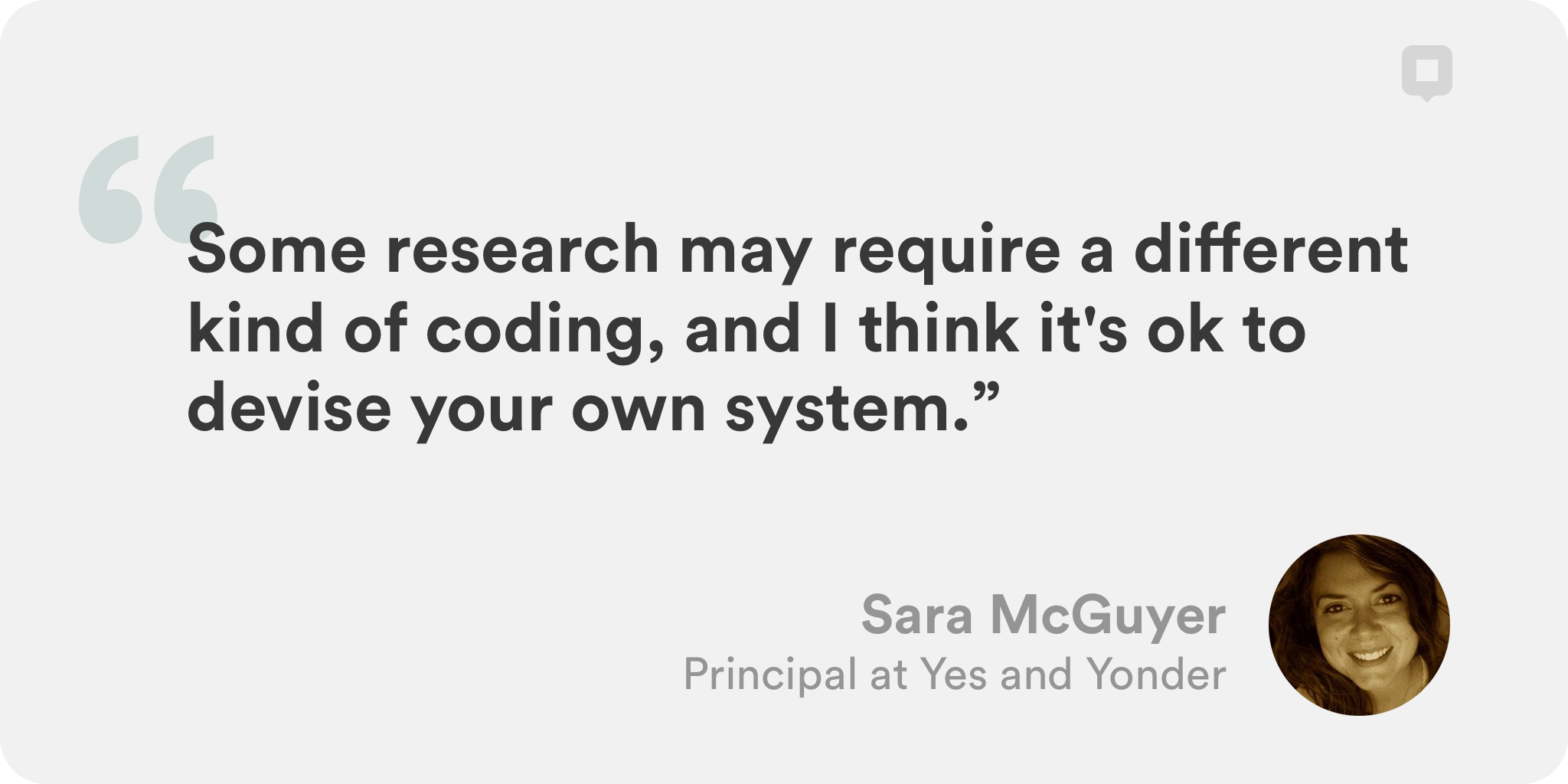
Developing a smart thematic tag list takes time, so don't be discouraged if you don't see a whole bunch of connections right away. The longer you sit with your data, the easier it will be to use thematic tags to quantify things like pain points, stages of a journey, different modes of behavior, or competing strategies.
Tagging can be a good tool to help flatten insights and communicate data to stakeholders—but it can also help teams understand data at a new level, Product and UX Designer Hannah Wei emphasizes.
“Because my end deliverables are snippets of insights shared with the product team, I try to strike a balance between presenting nuanced and actionable data,” Wei says. “I code for emotions to help my team empathize, and for motives and attitudes to help stakeholders build a mental model of their customers' needs.”
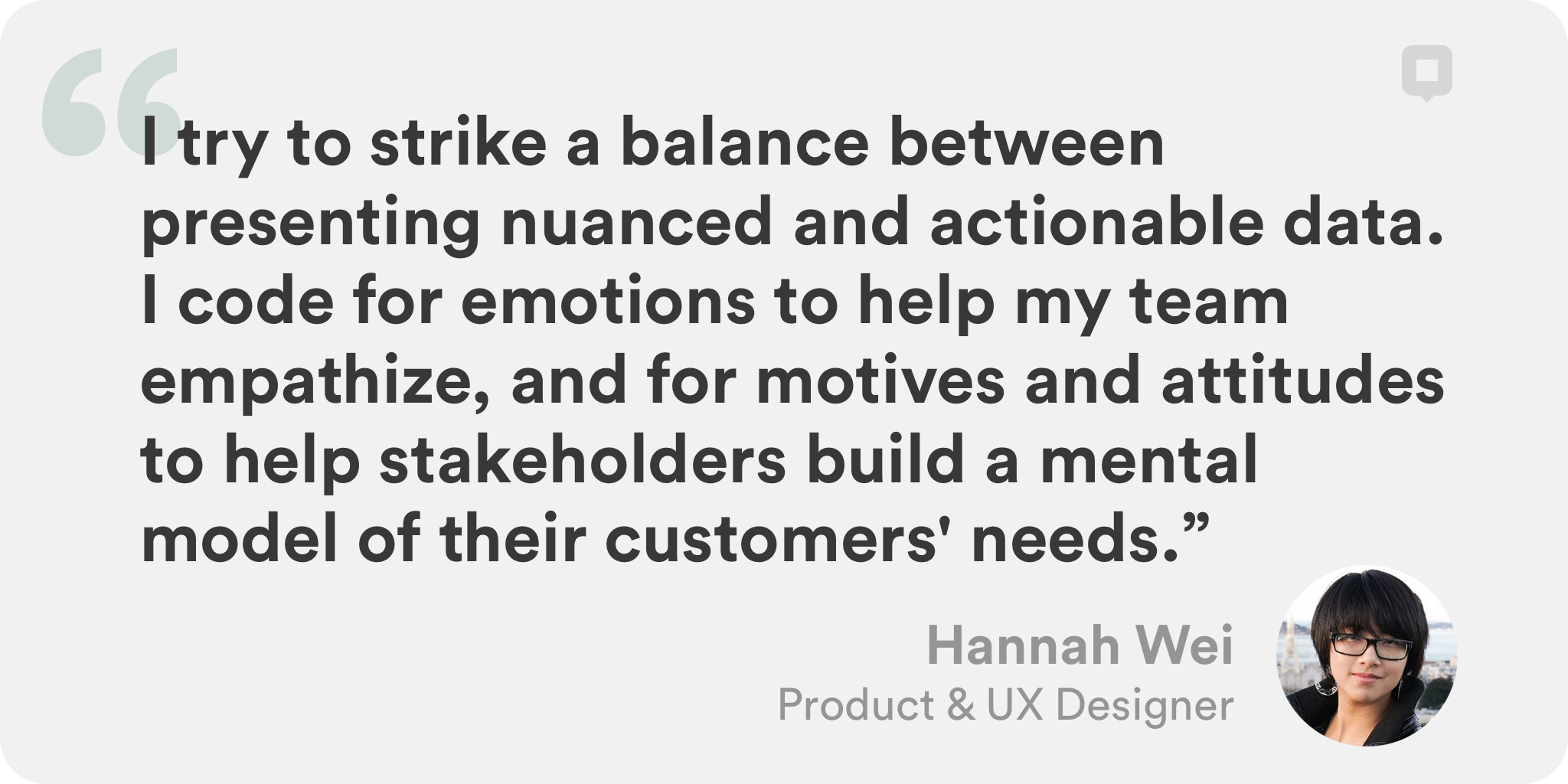
4. Remember that tags, like people, can evolve.
Don’t forget to revisit and rearrange tags in context down the line.
Jess Sand says her code groupings “sort of happen organically, if someone revisits a topic, or a theme starts to emerge as I’m transcribing/reading. The code gets refined as I continue through more interviews, and it’s not uncommon for me to rearrange sticky notes and change code terminology to accommodate new themes.”
And Hareth Al-Janabi advises that just because you coded something one way initially doesn’t mean there may be other angles worth revisiting. “Researchers need to remember to be inquisitive. We need to go beyond the words to get the most out of data. Think about things like the context in which the words were spoken, how the words link with what was said earlier (or later) and how it relates to what other participants have said about the topic.”
One tip is to apply your tags to 10-20% of your data, and then pause to evaluate how your tag list is working for you. Ask yourself: Does it feel difficult to apply your tags? Is your tag list mutually exclusive and comprehensively exhaustive? Are there any tags that overwhelm the rest of a data (or apply to 40% or more of your entries)? Are there any tags marked “other”? Are there any commonalities that you could use to create a new category?
Once you're confident that your tag list is right for your data set, you’re ready to dive into analysis.
If you’d like to see these analysis tips applied to research in dscout, stream our webinar about tagging to get quick, sharable insights.
Carrie Neill is a New York based writer, editor, design advocate, bookworm, travel fiend, dessert enthusiast, and a fan of People Nerds everywhere.

Subscribe To People Nerds
A weekly roundup of interviews, pro tips and original research designed for people who are interested in people