Iterative Study Designs: Why (and How) to Follow Up on Qual Research
Iterative studies are an incredible tool expanding on your insights. Dive into a handful of use cases and pull inspiration from our study on remote learning.
“If only we had thought to ask that” is a common regret in the research world. Perhaps you got surprising results on a quantitative question and you’re stuck theorizing why. Maybe you generated a new qualitative insight, but have no way of knowing just how relevant it is to your user base as a whole.
It’s those moments that leave us kicking ourselves. There’s potentially a great insight in our data, but we have to leave it on the table because we just don’t have enough information!
Once this has happened enough times, research design can start to feel stressful.
We want to throw everything at the wall and hope that something sticks, for fear of regretting that we didn’t ask that one question. Or, we agonize over the wording of a new question, not knowing exactly how participants are going to connect with it.
This alone makes the case for iterative research—running smaller back-to-back research initiatives, instead of over-indexing on one, over-scoped study.
The stress of design or the regret of analysis dissipates when you can have multiple shots at uncovering an insight. Iterative research design gives you the freedom to be more creative with your approach. When you’re no longer wasting your “one shot” at research, you can try more “wacky” approaches, sometimes with unexpected and fantastic results.
Iterating can also give you the chance to pivot your research to where the actually interesting insights are, and abandon the threads that aren’t as fruitful. You can pilot a new question or design to see if it’s going to work, ensuring that you’re going to get what you need when you ultimately fire off your full-scale research effort.
Jump to a section:
Four instances that call for iterative study designs
There are quite a few ways you could use dscout to iterate on study design, depending on what you hope to accomplish with an iteration. Here are a few cases that come to mind when iterating, and what you might want to do to accomplish them:
“I want to explore”
Sometimes iteration is just about wondering! You’re not quite sure what’s interesting yet, so you ask a few questions and see what thoughts they generate. Then, you ask a few more until you strike insight gold.
A few ways to start structuring your exploration in dscout (or your qual research tool of choice):
- Express missions/media-rich surveys. These are a fantastic low-lift way to get quick answers and do initial exploration in your space. A typical mission for us consists of a 5-10 question survey, a smaller sample size (20-40 people) and a timeframe of 24 hours. These allow you to experiment and converse with your team on a rolling basis and follow any thread that seems interesting. Missions like these are best used for informal and exploratory conversations.
- Diary mission/longitudinal study. If anything you discover from your initial survey starts seriously piquing your interest, you can take what you’ve learned to inform a more involved study that dives deeper. For us, this generally means a longitudinal study on Diary—getting a sense of whether the behaviors we saw in our initial Express mission are trends that appear in-context and over-time.
- Live mission/1:1 interviews: Still not enough data? Follow up with in-depth interviews for a deeper dive.
This is a lightweight, standard, mixed-methods approach, that should allow you to layer your data and triangulate insights within it. This iterative method starts quant and ends qual.
“I want to pilot”
If you have a creative research idea but are unsure if it’s going to work, try launching a “beta” research design. There are a number of ways you can do this with Express or a short survey:
- Start broad, then narrow down the questions. Launch specific questions to a broad population and see if the responses you’re getting back are aligned with the data you need. Then, investigate them further with a larger study.
- A/B test. Run two quick-turn studies concurrently and A/B test the different question types or wordings. With dscout, you can run two similar Express missions, housed within different “projects” to easily accomplish this.
- Survey after a Diary mission. You can also pilot 1-2 different parts in a longer dscout Diary mission with a small sample (n=10ish). Then, add an extra part asking participants what they thought about completing the survey. You could replicate this design with iterative surveys sent to the same audience.
These examples are small scale to large scale, and usually part to whole, but don’t belong in a specific method style.
“I wish I had asked that question”
If you didn’t ask a key follow up question during your study, here are a few ways to get some additional context:
- Launch a “$0.50 Express mission” (or other, low-lift survey). If you found a surprising quantitative answer in your larger study but didn’t ask a follow-up, launch a quick $0.50 Express mission that repeats a single quant question and then asks a follow-up about it. If you need the same participants, launch a restricted Express to the same participant group you used for your diary study.
- Plan for two studies. Write an Express mission that only asks quantitative questions with the intention of launching a second mission that follows up on the most interesting findings. This avoids wasting money and user time on any follow-ups questions that aren’t that interesting for your team.
- Launch a targeted, deliverable-focused follow up. If you have an interesting insight and want to add visual data, like videos or pictures, use a limited, targeted Express mission to collect that supporting data.
“I want to validate or quantify this”
If you’re looking to dig into an interesting response to see if it has legs as an actual insight or is merely a singular opinion, turn your qualitative findings into mixed-method findings. This provides an opportunity to ground your small-scale results in large-scale frequency data to see just how prevalent they might be in your customer base.
To do this, consider the following approaches for your “follow-up” study design:
- Turn your first study’s themes into likert questions/picklists. If you’re finding interesting themes in an in-depth interview, or a qual-heavy diary study, turn them into likert questions or picklists (see details below in the design section). Run them in an Express mission or survey to see which ones resonate with a larger crowd.
- Ask follow-up media or open-ends to follow up from your first study’s closed-ended questions. Look for quick hit story data or emotion data that supports your initial findings.
- Get cleaner data than your first study afforded you. Sometimes you use an open-end because you’re not quite sure what you need to ask. If the answers get you murky insights, you can crystallize the open-end into a closed-end and get better, clearer responses.
- Try a larger participant pool than your first study. Often, you need a lot more participants to get valid quant than to get valid qual. This will break up those two needs, so you can design studies that cater to your budget and timeline.
These examples start with qual and small-scale, and end as quant and large-scale.
How we design iterative studies
Express is by far the most nimble way of making sequential research that dscout has to offer—so we rely on it for most iterative studies. However, if you don't have a dscout subscription, these principles can be applied to surveys or unmoderated studies on your tool of choice.
Knowing the quick-turn, low-lift nature of Express, we felt that we had a lot of freedom to throw some "weird" questions at the wall. We didn’t feel beholden to understanding whether insights were “real” or not right away; we were really looking for anything that might be interesting. There was flexibility to keep the mission very small, because data validation would come later down the line.
Some things to keep in mind as you’re designing your own iterative study:
- Even though it’s a qualitative study, you should still include some quant questions to prime your scouts and help your analysis down the road.
- Think of some of the qualitative questions as reverse-engineered follow-ups. For example, “If this were more mixed-methods, what quantitative finding would this answer help explain?”
- If you want to quantify certain qualitative answers, make sure your questions are streamlined enough to allow for easy coding. For example, instead of asking, “What are all of the things you like about this product?” ask, “What’s one thing you like about this product?” or, “What’s your favorite thing about this product?” It will make the responses easier to sort through. Plus, when you have quantified themes, you’ll have great quotes that can speak to one idea at a time.
- Don’t be afraid to get a little weird with it! This is just a first draft and it’s ok if you’re not quite sure what you’re going to get out of a question.
Analyzing the first study
Keep in mind, this analysis isn’t about finding insights -- it’s about finding more questions.
Start by immersing yourself and having conversations with your team. The analysis doesn’t need to be very formalized at this stage. You’re looking for anything that makes you go, “That’s interesting,” or any quotes that make you think, “If this was a common sentiment, that would be very powerful.”
Try to think towards your deliverable. If you see great quotes in your data, ask yourself, “Would I want to see a graph alongside that quote?” What graph are you envisioning? That’s your new question!
Then, look at thematic open-ends and try to solidify the main themes coming through. There’s no need to tag because there’s no need to quantify 30 open-ends when you’re taking your findings to a larger scale. Focus on impressionistically choosing the top 5-10 themes you’re seeing and try to crystallize them into an easily digestible list.
Tip: you can use the dscout platform to generate word clouds, which can be a shortcut to generating picklists around scout sentiments!
Designing your follow-up study
Where the first design can be free-wheeling, this is where you need to rein it in a bit. The key word here is follow-up–ask questions that help you better understand the insights you began to uncover in the first mission. If you start asking brand new questions, you may end up getting stuck in an iteration cycle that will always leave you with unanswered questions.
Another important consideration here is scale. The bigger and more quant-minded you want this second mission to be, the more you should avoid asking follow-up qual questions. These will raise the price on your mission, which can balloon quickly at larger sample sizes. Keep it lean and keep it quant–try to rely on your first mission to get the qualitative color you need.
Areas to focus on:
- Quantifying themes using picklists derived from open ends from the first study
- Scaling quantitative emotion questions or Likert questions that were either asked in the first study or derived from the first study findings
- Asking for word clouds or emotion pick-lists to understand the most resonant sentiments from your small-scale work
An ideal study here is short and lean to get maximum engagement. Afterwards, feel free to open it up to a much larger audience.
Analyzing your findings
Now that you have two sides of your research story, you can start putting it all together. You’ve likely developed some hunches from your qualitative run, which were (hopefully) confirmed by your new quantitative backing. This should make final analysis relatively straightforward.
Go back into your first study and identify what you now know to be the most important or common themes, tag if necessary, and drill down into those areas to find the quotes or artifacts that you need.
Presenting your iterative study findings won’t be much different than presenting a single large-scale mixed-methods survey. Use your qualitative findings to color your well-formed quantitative questions, or use your quantitative work to legitimize and bolster the insight you intuited out of the smaller study.
Plus, the iteration doesn’t need to stop here! If you’ve used this process to land on a fascinating insight and you or your stakeholders must know more, you can treat this as a pilot for a multi-part mixed methods survey in the diary tool!
Example iterative design: Remote learning
We wanted to know what remote learning has been like for high-schoolers and college students during the pandemic. We weren’t sure exactly what to ask a wide range of kids to get a proper pulse on the experience, so we started with a qual-focus express mission aimed at a small sample (n=30).
Questions we asked:
- Take a picture of your remote learning workspace. If you work in multiple places, take a picture of the space you use most often.
- Tell us about your workspace! What do you like about it? What do you wish was different?
- What’s one BENEFIT to remote education, if any?
- What’s one DRAWBACK to remote education, if any?
- Why do you feel more or less happy in a remote setting compared to in-person learning?
- Why do you feel more or less productive in a remote setting compared to in-person learning?
- This is your last question! What’s one thing you wish you could tell teachers about remote learning?
After the results were back, we dug in and found a few interesting things. This led to some questions for us:
- We noticed from the pictures and descriptions of workspaces that a lot of the participants were doing their work in their beds. We hadn’t considered this, because as professionals we’ve prioritized getting a desk for ourselves (and had the funds and agency to do so). A blind spot on our part!
Now we wondered: Exactly how common is it for college students to work from bed? How many of them have a space of their own for work? - Scouts had a lot to say about benefits and drawbacks, but no one or two themes were emerging clearly enough. We wanted to know which of these was really the most prevalent.
Now we wondered: Which benefits and drawbacks are top of mind for the larger student population? - We also noticed that motivation was coming up as a commonality. This was separate from productivity, which made us want to capture that metric as well.
Now we wondered: Scouts say they’re relatively productive, but does that really mean they’re motivated? Or are they overcoming a lack of motivation? - Scouts wished their teachers understood how difficult the remote learning process can be.
Now we wondered: What’s the emotional landscape of students in remote learning?
We used our second survey to fill in the blanks and validate existing data. Our sample size was much larger (n=500). We kept the survey short and inexpensive (12 questions, $1 per complete) to make it as broadly accessible as possible.
Some close-ended questions (multiple choice/single select options) we included were :
- Where in your home do you normally do your remote learning work? If you work in multiple places, select the place you work most often.
- List three words that you think best describe the experience of remote learning.
- How would you compare your *productivity* in remote learning compared to in-person learning?
- How would you compare your *motivation* in remote learning compared to in-person learning?
- How would you compare your *happiness* in remote learning compared to in-person learning?
- Out of the following list, which do you think is the *biggest benefit* to remote learning?
- I don’t have to commute
- I get more sleep
- I save money
- I don’t have to attend lectures
- Notes, PowerPoints, and other resources are more available
- I get to work at my own pace
- I’m more comfortable
- I have a more flexible schedule
- I get to eat whatever I want
- It’s easier to focus
- Other (tap to type)
- Out of the following list, which do you think is the *biggest drawback* to remote learning?
- I can’t see my friends
- I don’t get to know my classmates or community
- I can’t ask as many questions or attend office hours
- Collaborating with my peers in class is difficult
- I can’t do hands-on lessons or assignments
- I get distracted easily
- Technical issues get in the way of learning
- My remote learning equipment isn’t good enough
- I can’t properly manage my time
- My health is impacted (e.g. back problems, eye strain, etc.)
- Classes are more boring
- Other (tap to type)
- Please choose three emotions that you most associate with remote learning from the following list.
- Anger
- Exasperation
- Irritation
- Fear
- Nervousness
- Joy
- Contentment
- Optimism
- Pride
- Sadness
- Disappointment
- Neglect
- Sadness
- Shame
- Surprise
(Bonus!) Insights pulled from our studies
From quant study, we learned that 76% of students feel less motivated in remote learning, and 72% feel less productive. The top drawback of remote learning is that they’re distracted more easily.
We get qualitative color and nuance about distraction and motivation from the qual study:
"When I’m in school I have set times to work on my school work and set times for breaks and lunch, but at home I kind of just do everything whenever I feel like it and it took me off my normal schedule so I don’t get as much done."
"It is easy to get distracted, and I enjoy seeing my professor daily, it helps keep me accountable to my schoolwork, and staying on top of things."
"One drawback to remote education is the lack of motivation I experience. I am a student who gets straight As and has a perfect gpa, but I still struggle with having the willpower to say no to being on my phone all day instead of doing work."
From quant study, we learned that 58% of students feel less happy in remote learning. Missing friends and the community of the classroom are both in the top five drawbacks of remote engagement.
From qual study, we learn more what loneliness means to people:
"I was less happy in a remote setting because I was no longer able to spend time with my friends. The interpersonal relationships you build with your peers are so important to me and overnight it was gone. Once the pandemic started, I never saw my friends again and I have since graduated."
"I think happiness stems from being around other people, and I’m not doing that when I am learning in a remote setting. I miss my peers!"
Our survey of workspace styles revealed that the top workspace is a bedroom desk (49%) and (confirming our hunch) the second most common is students’ beds (35%).
From our qual study, we collected some cool artifacts and quotes about people’s workspaces:
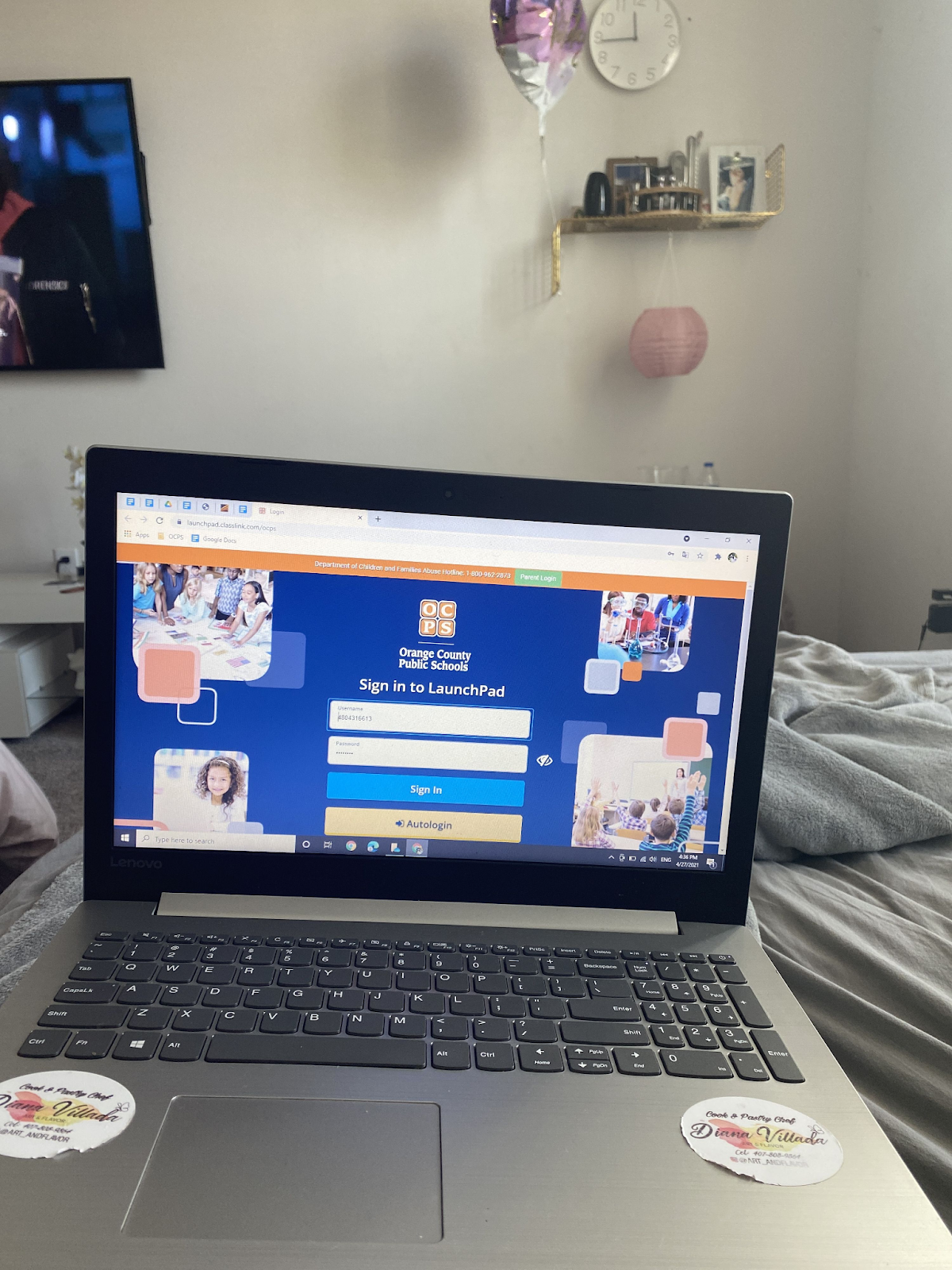
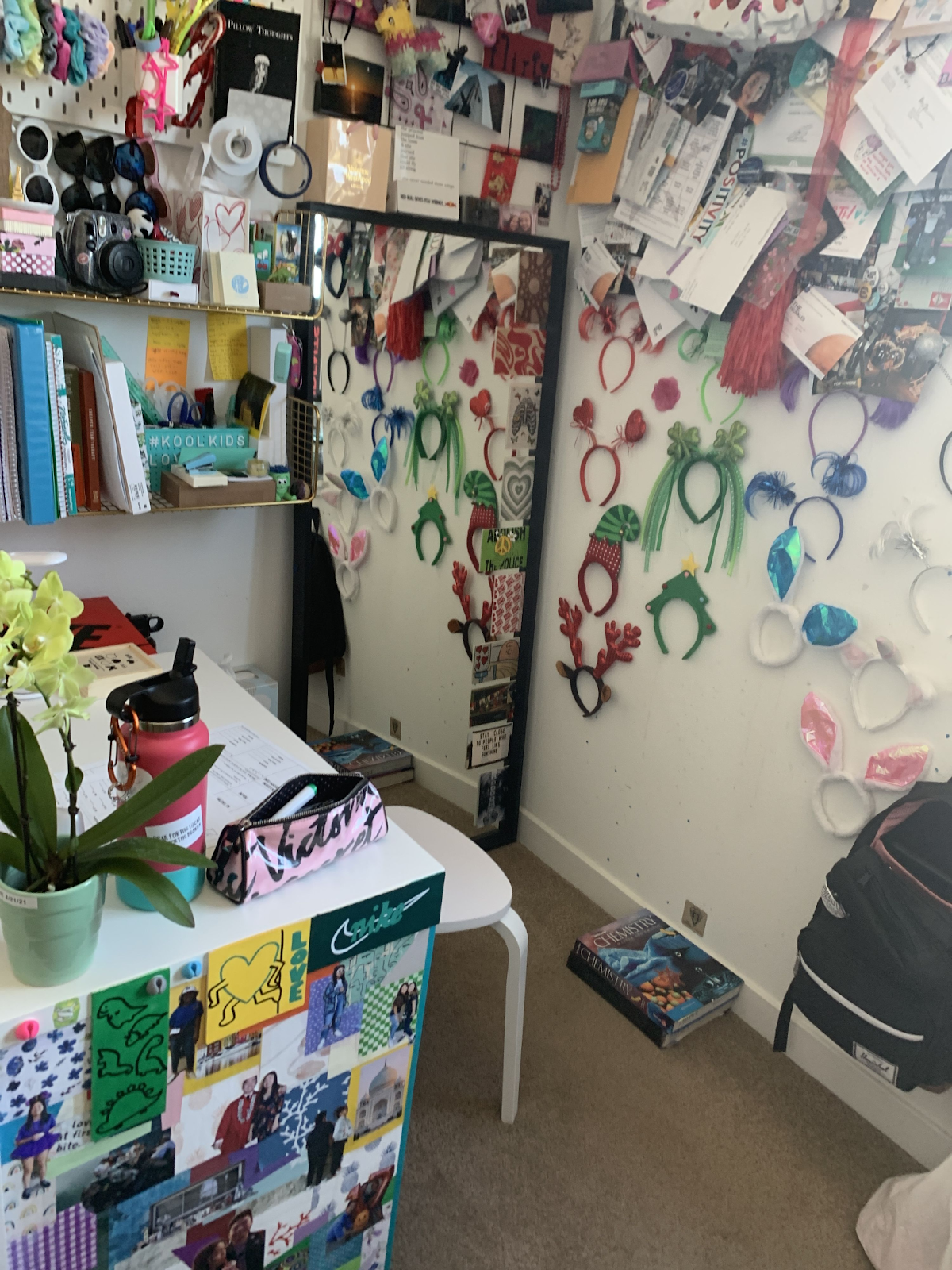
Karen is a researcher at dscout. She has a master’s degree in linguistics and loves learning about how people communicate with each other. Her specialty is in gender representation in children’s media, and she’ll talk your ear off about Disney Princesses if given half the chance.

Subscribe To People Nerds
A weekly roundup of interviews, pro tips and original research designed for people who are interested in people